LM Inspect
LM Inspect is a software program that offers a capability to perform automated inspection. For example, this software is applicable to the whole surface inspection of small defects and particles on wafers and glass substrates. The program also enables users to review defects chosen on a defect map and/or to classify them, for example, by size, color (blank or white), or by type (pit or bump).
The operation screen of LM Inspect is shown in the picture below. You simply set a sample and start operation by following the measurement flow. Inspection proceeds automatically. Once it ends, the results (review image, classification, and defect map) will be shown on the screen automatically.
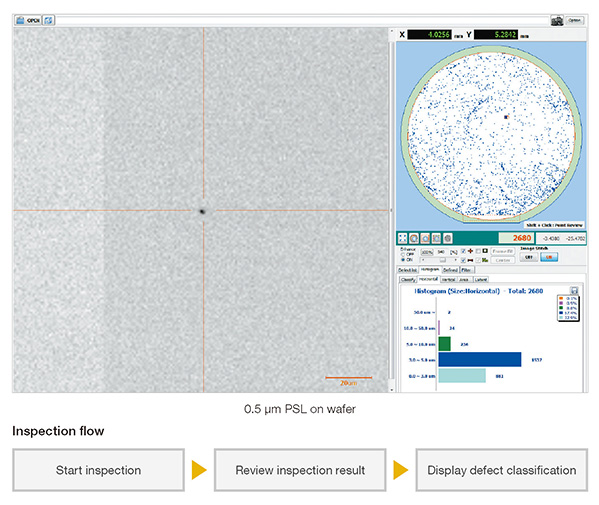
Shown below is an example of SiC wafer inspection. With confocal and differential interference used in combination, HYBRID+ detects defects that scatter light with low intensity and captures high resolution images. It allows users to conduct defect review and defect tracing afterwards. By using the optional Haze Map function, you can visualize surface roughness as well.
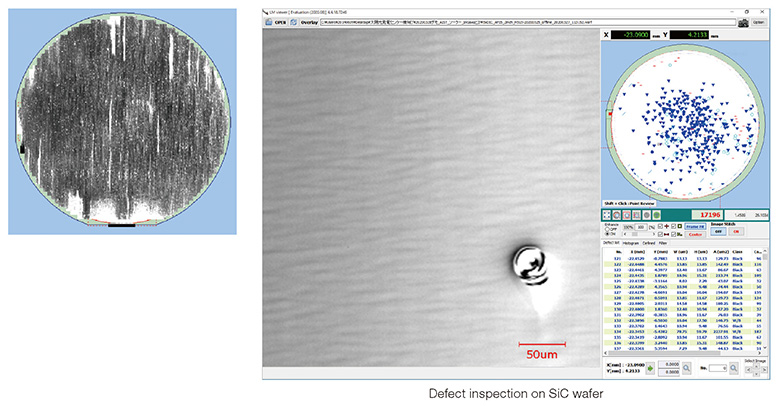
LM Brain
LM Brain is an optional software program that uses artificial intelligence for inspection and classification. It enables users to perform inspection on difficult samples such as repeated patterns and random patterns. LM Brain has enhanced its capability to perform highly accurate defect classification by adopting Deep Learning.
Defect inspection based on pattern comparison
HYBRID+ has enhanced its capability to enable the performance of inspection on a patterned sample and a sample with a repeated pattern once you register the images of a "good" sample without defect.
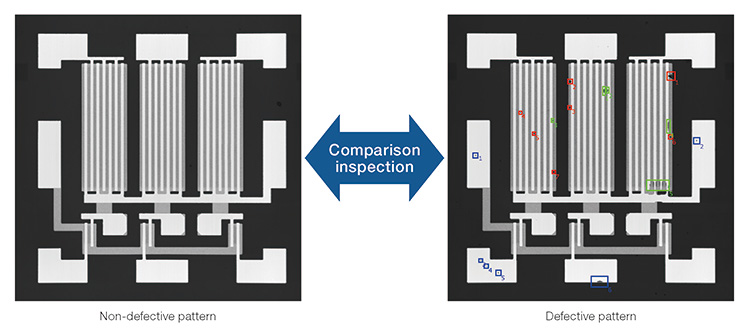
Defect classification by Deep Learning
Deep Learning identifies killer defects that affect device performance among defects detected by HYBRID+. It is capable of classifying defects that are otherwise difficult to classify.
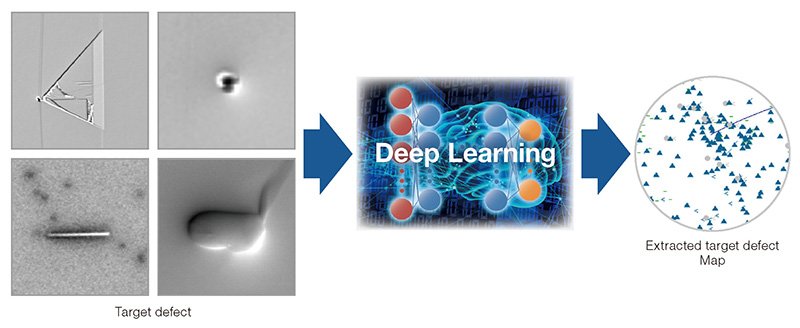
You might also be interested in